GARY CHANG, MASc
Predictive Modelling of Stack Effect Using Machine Learning Algorithms in High-Rise Office Buildings
Read the full Master Research Paper HERE.
Stack effect adversely affects heating loads in high-rise buildings due to excess infiltration caused by pressure differentials. Mitigating the effects of this phenomenon is crucial to meet the increasingly ambitious energy targets such as Canada’s commitment to COP21. A novel data- driven modeling approach is implemented in order to classify instances where stack effect is detrimental to building performance based on the pressure differentials, building automation system (BAS), and weather data. Various machine learning algorithms were tested with K- nearest neighbor (KNN) achieving the best results for stack effect detection via pressure differentials with an accuracy of 97.7%. The research also investigated potential methods of simplifying stack effect detection by using BAS data as a proxy variable with the best model being able to correctly classify 77.5% of the instances during the cooling season.
Predictive Modelling of Stack Effect Using Machine Learning Algorithms in High-Rise Office Buildings
Read the full Master Research Paper HERE.
Stack effect adversely affects heating loads in high-rise buildings due to excess infiltration caused by pressure differentials. Mitigating the effects of this phenomenon is crucial to meet the increasingly ambitious energy targets such as Canada’s commitment to COP21. A novel data- driven modeling approach is implemented in order to classify instances where stack effect is detrimental to building performance based on the pressure differentials, building automation system (BAS), and weather data. Various machine learning algorithms were tested with K- nearest neighbor (KNN) achieving the best results for stack effect detection via pressure differentials with an accuracy of 97.7%. The research also investigated potential methods of simplifying stack effect detection by using BAS data as a proxy variable with the best model being able to correctly classify 77.5% of the instances during the cooling season.
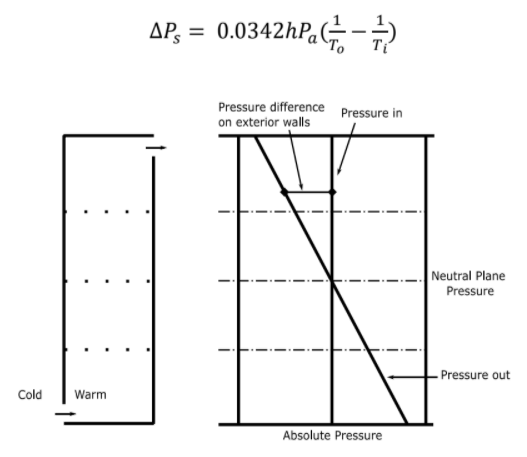