BUILDING SCIENCE
Javeriya Hasan—Alumni Research Award
![]()
Javeriya Hasan—Alumni Research Award
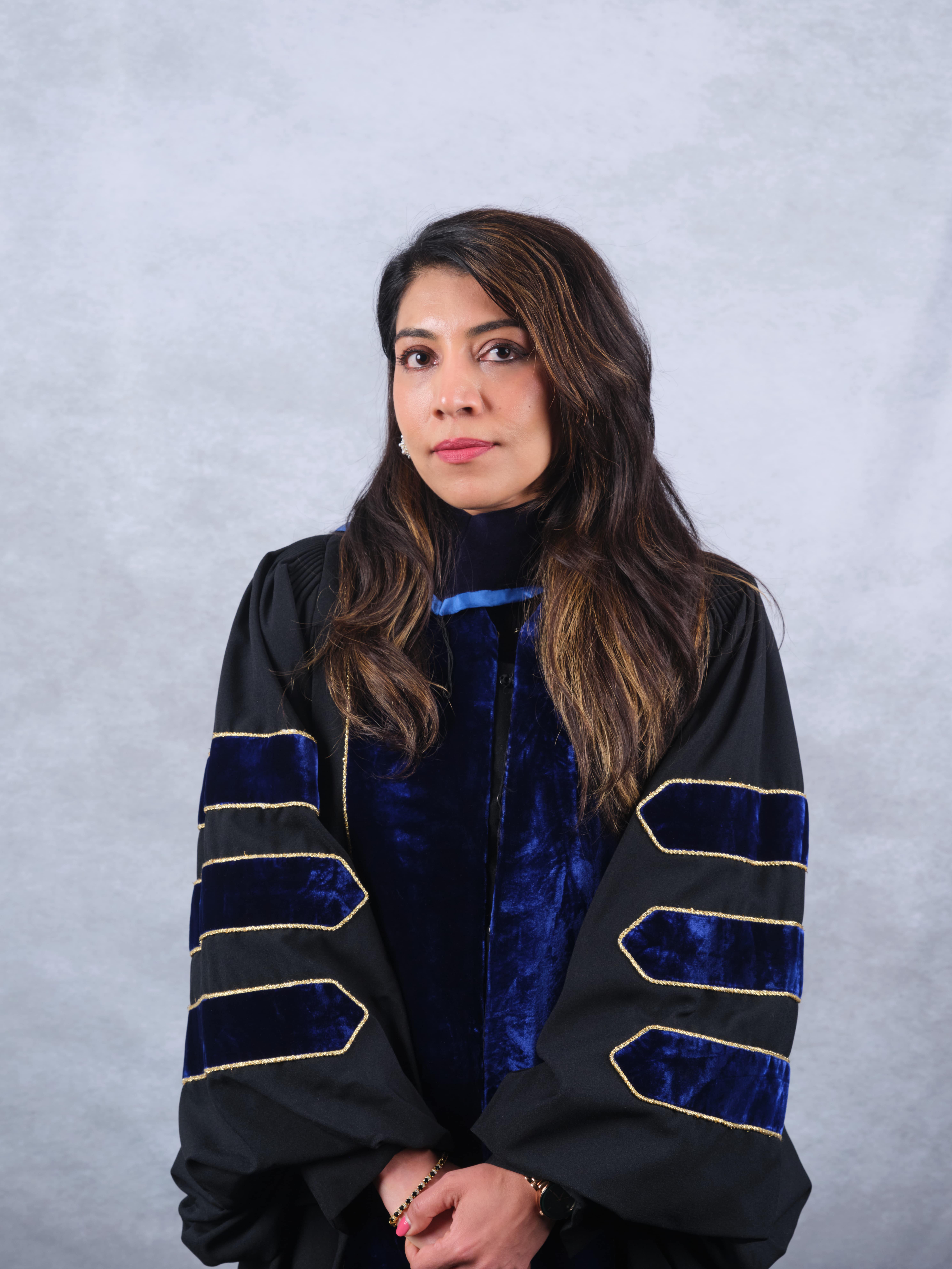
About the Award
For overall achievement, contribution, and commitment to the Building Science Graduate Program.
My PhD research focused on the importance of controlled densification in urbanized cities, developing a framework for a tool on solar neighbourhood design. In the context of energy resilience and self-sufficiency, it’s important to have solar access in dense urban areas as it enables passive heating, natural lighting, and on-site solar power, reducing energy demands. Rooftop photovoltaics alone could meet up to 33% of global urban electricity needs, demonstrating the significance of solar accessibility in urban neighbourhoods. In Toronto, where rapid growth poses a challenge, city guidelines like the Toronto Green Standard and Mid-Rise Guidelines promote solar-ready buildings and sidewalk solar access, but could further provisions for comprehensive neighbourhood solar access. ‘Solar Envelopes,’ adapted to Toronto’s unique neighbourhoods, offer a solution to maintain solar access amidst the densification taking place in the city.
The research objective was to shift from a simulation-based approach to a data-centric tool that simplifies early-stage building design decision-making governing solar access in existing neighbourhoods. Traditional simulation methods are computationally intensive, costly, and time-consuming, often requiring specialized skillsets.
The methodology used in this research involved a structured approach: identifying suitable neighbourhoods situated along arterial roads in Toronto; architectural simulations involving solar access evaluations of 3D neighbourhood models, followed by identifying and extracting relevant data, then representing spatial and temporal data and target metrics to populate a comprehensive dataset, and finally, developing machine learning (ML) models. This methodology enabled a nuanced understanding of how parameters governing neighbourhood morphology influenced solar access in the existing neighbourhood through a ‘polar locus’ approach applied to represent building attributes and positions in terms of spatial parameters. While existing Solar Envelope research often relies on tools like Rhino/Grasshopper, integrating ML offered enhanced predictive accuracy, allowing for comprehensve insights on the diverse neighbourhoods investigated. Although Toronto served as the case study, the methodological approach would apply to other global cities experiencing similar urban growth as they could also benefit from exploring data- drive approach regarding Solar Envelopes to sustain solar access amidst densification.
By using a data-driven approach, the tool that that has culminated from this PhD research enables early-stage decision-making for architects and urban planners, predicting allowable building heights based on neighbourhood spatial characteristics and solar access needs.
The study identified specific neighbourhood features, such as rooftop area and facade height in certain orientations, that significantly influence solar access and inform the tool’s predictive model. The most effective ML model achieved satisfactory accuracy, particularly in mid-rise neighbourhoods.
For overall achievement, contribution, and commitment to the Building Science Graduate Program.
My PhD research focused on the importance of controlled densification in urbanized cities, developing a framework for a tool on solar neighbourhood design. In the context of energy resilience and self-sufficiency, it’s important to have solar access in dense urban areas as it enables passive heating, natural lighting, and on-site solar power, reducing energy demands. Rooftop photovoltaics alone could meet up to 33% of global urban electricity needs, demonstrating the significance of solar accessibility in urban neighbourhoods. In Toronto, where rapid growth poses a challenge, city guidelines like the Toronto Green Standard and Mid-Rise Guidelines promote solar-ready buildings and sidewalk solar access, but could further provisions for comprehensive neighbourhood solar access. ‘Solar Envelopes,’ adapted to Toronto’s unique neighbourhoods, offer a solution to maintain solar access amidst the densification taking place in the city.
The research objective was to shift from a simulation-based approach to a data-centric tool that simplifies early-stage building design decision-making governing solar access in existing neighbourhoods. Traditional simulation methods are computationally intensive, costly, and time-consuming, often requiring specialized skillsets.
The methodology used in this research involved a structured approach: identifying suitable neighbourhoods situated along arterial roads in Toronto; architectural simulations involving solar access evaluations of 3D neighbourhood models, followed by identifying and extracting relevant data, then representing spatial and temporal data and target metrics to populate a comprehensive dataset, and finally, developing machine learning (ML) models. This methodology enabled a nuanced understanding of how parameters governing neighbourhood morphology influenced solar access in the existing neighbourhood through a ‘polar locus’ approach applied to represent building attributes and positions in terms of spatial parameters. While existing Solar Envelope research often relies on tools like Rhino/Grasshopper, integrating ML offered enhanced predictive accuracy, allowing for comprehensve insights on the diverse neighbourhoods investigated. Although Toronto served as the case study, the methodological approach would apply to other global cities experiencing similar urban growth as they could also benefit from exploring data- drive approach regarding Solar Envelopes to sustain solar access amidst densification.
By using a data-driven approach, the tool that that has culminated from this PhD research enables early-stage decision-making for architects and urban planners, predicting allowable building heights based on neighbourhood spatial characteristics and solar access needs.
The study identified specific neighbourhood features, such as rooftop area and facade height in certain orientations, that significantly influence solar access and inform the tool’s predictive model. The most effective ML model achieved satisfactory accuracy, particularly in mid-rise neighbourhoods.
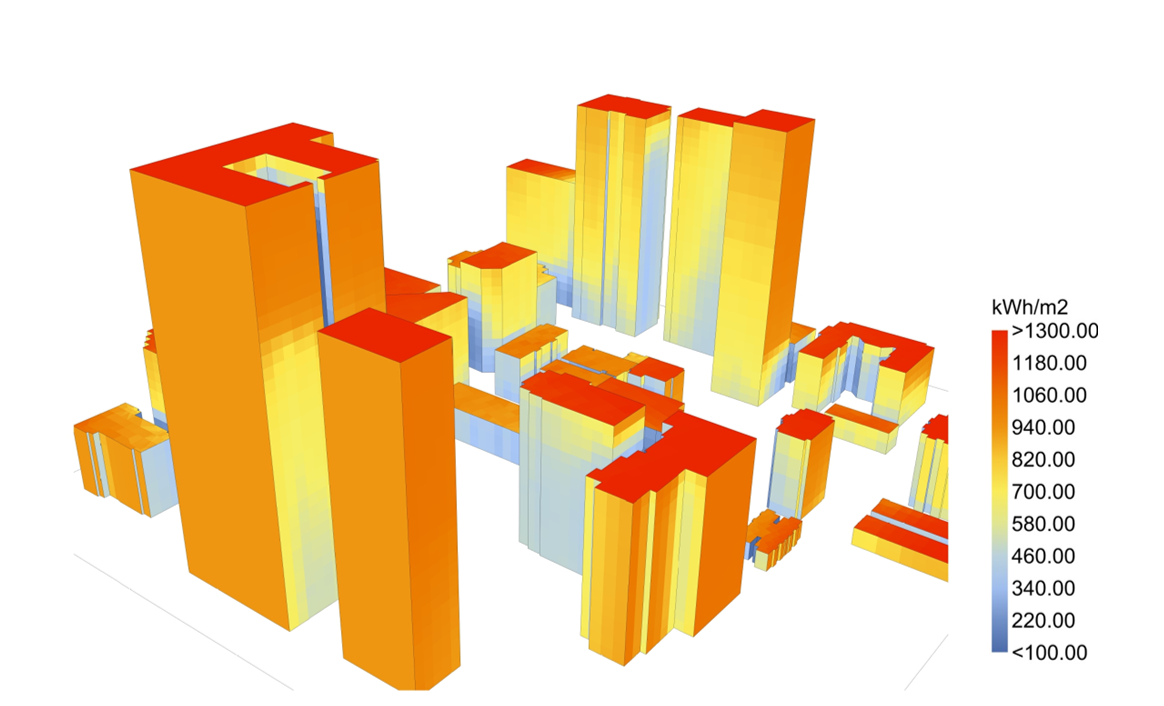
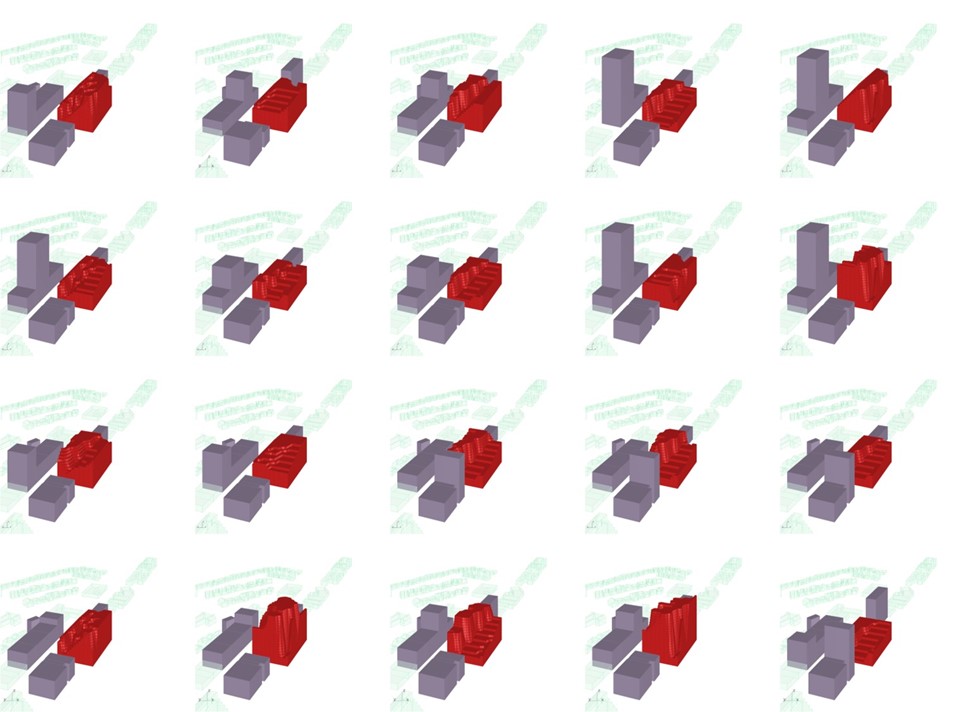
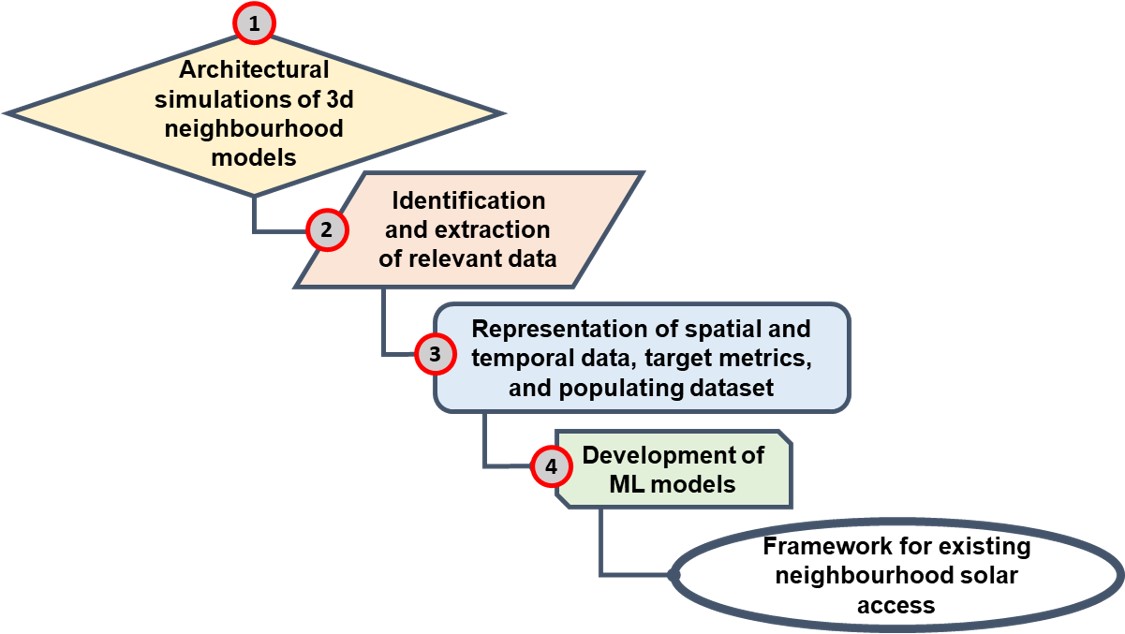
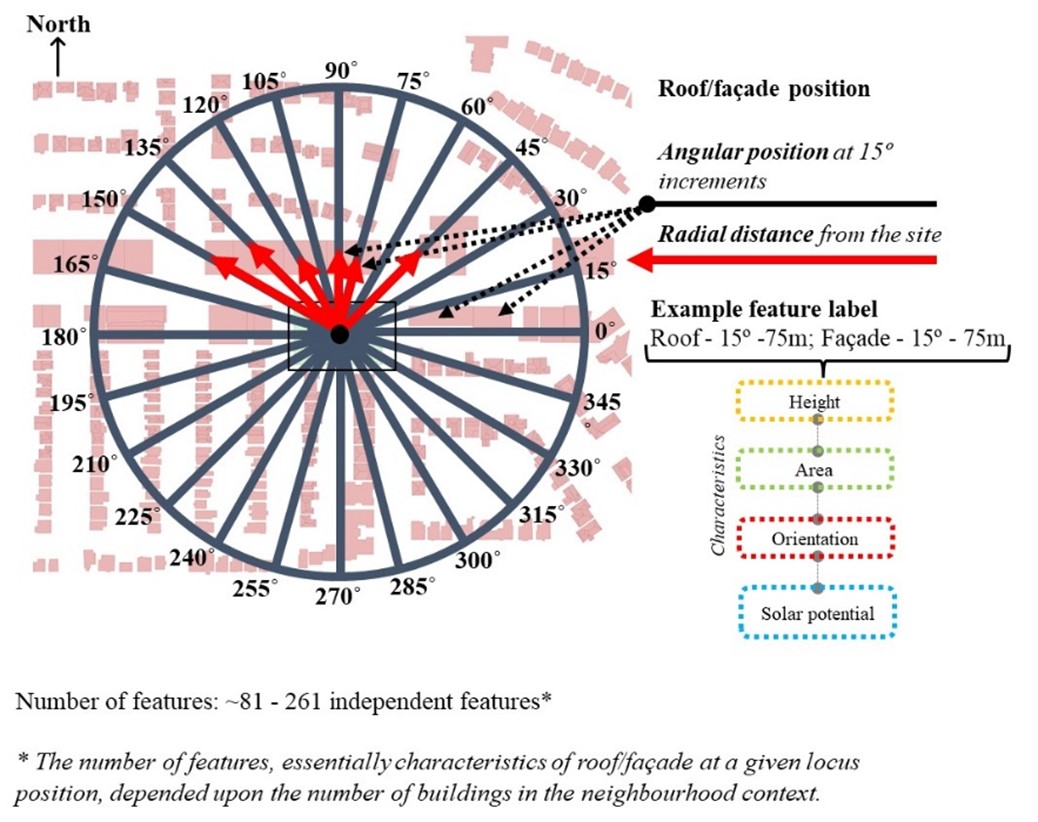